M. Sc. Stephan Vogt
Team Leader: Autonomous Energy Systems (AESy)
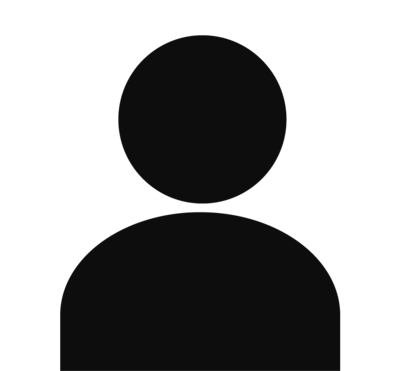
- Fax
- +49 561 804-6022
- stephan.vogt[at]uni-kassel[dot]de
Publications
2024[ to top ]
- PrOuD: Probabilistic Outlier Detection Solution for Time Series Analysis on Real-world Photovoltaic Inverters. Energies (MDPI). 17, 64 (2024). https://doi.org/10.3390/en17010064.
2023[ to top ]
- Targeted Adversarial Attacks on Wind Power Forecasts. Machine Learning. 113, 863–889 (2023). https://doi.org/10.1007/s10994-023-06396-9.
- Power flow forecasts at transmission grid nodes using Graph Neural Networks. Energy and AI. 14, 100262 (2023). https://doi.org/10.1016/j.egyai.2023.100262.
- Multi-Task Representation Learning for Renewable-Power Forecasting: A Comparative Analysis of Unified Autoencoder Variants and Task-Embedding Dimensions. Machine Learning and Knowledge Extraction (MAKE). 5, 1214–1233 (2023). https://doi.org/10.3390/make5030062.
2022[ to top ]
- Unified Autoencoder with Task Embeddings for Multi-Task Learning in Renewable Power Forecasting. In: International Conference on Machine Learning and Applications (ICMLA). bll 1530–1536. IEEE (2022). https://doi.org/10.1109/ICMLA55696.2022.00240.
- Fast Bayesian Updates for Deep Learning with a Use Case in Active Learning. arXiv e-prints. arXiv:2210.06112 (2022).
2021[ to top ]
- Uncertainty and Utility Sampling with Pre-Clustering. In: Workshop on Interactive Adaptive Learning (IAL), ECML PKDD (2021).
- The Problem with Real-World Novelty Detection -- Issues in Multivariate Probabilistic Models. In: Workshop on Self-Improving System Integration (SISSY), ACSOS. bll 204–209. IEEE (2021). https://doi.org/10.1109/acsos-c52956.2021.00055.
- Task Embedding Temporal Convolution Networks for Transfer Learning Problems in Renewable Power Time Series Forecast. In: European Conference on Machine Learning and Knowledge Discovery in Databases (ECML PKDD): Applied Data Science Track. bll 118–134. Springer (2021). https://doi.org/10.1007/978-3-030-86514-6_8.
2020[ to top ]
- Statistical correction scheme for the wind power allocation to transformer stations in the transmission grid. Electric Power Systems Research. 189, 106623 (2020). https://doi.org/10.1016/j.epsr.2020.106623.
- Quantile Surfaces -- Generalizing Quantile Regression to Multivariate Targets. arXiv e-prints. arXiv:2010.05898 (2020).
- Evaluation of Anomaly Detection of an Autoencoder Based on Maintenace Information and Scada-Data. Energies. 13, 1063 (2020). https://doi.org/10.3390/en13051063.
2019[ to top ]
- Wind Power Forecasting Based on Deep Neural Networks and Transfer Learning. In: Wind Integration Workshop. , Dublin, Ireland (2019). https://doi.org/10.24406/publica-fhg-406413.
- Deep Learning Based Failure Prediction in Wind Turbines Using SCADA Data. In: Conference for Wind Power Drives (CWD). bll 391–404 (2019).
- Bayesian parameterisation of a regional photovoltaic model--Application to forecasting. Solar Energy. 188, 760–774 (2019). https://doi.org/10.1016/j.solener.2019.06.053.
2018[ to top ]
- Multi-task distribution learning approach to anomaly detection of operational states of wind turbines. In: Journal of Physics: Conference Series. bl 012040 (2018). https://doi.org/10.1088/1742-6596/1102/1/012040.
- Benchmark of Spatio-temporal Shortest-Term Wind Power Forecast Models. In: Wind Integration Workshop (2018).
- A review of uncertainty representations and metaverification of uncertainty assessment techniques for renewable energies. Renewable and Sustainable Energy Reviews. 96, 352–379 (2018). https://doi.org/10.1016/j.rser.2018.07.042.
2017[ to top ]
- Application of numerical weather prediction in wind power forecasting: Assessment of the diurnal cycle. Meteorologische Zeitschrift. 26, 319–331 (2017). https://doi.org/10.1127/metz/2017/0820.
2016[ to top ]
- A Review of Deterministic Error Scores and Normalization Techniques for Power Forecasting Algorithms. In: IEEE Symposium Series on Computational Intelligence (SSCI). bll 1–9. IEEE, Athens, Greece (2016). https://doi.org/10.1109/ssci.2016.7849848.
2015[ to top ]
- An empirical approach to parameterizing photovoltaic plants for power forecasting and simulation. Solar Energy. 120, 479–493 (2015). https://doi.org/10.1016/j.solener.2015.07.024.