Workshops
Lecturer: Prof. Sandra S. Rossie (Perdue University, Indiana; https://ag.purdue.edu/biochem/Pages/profile.aspx?strAlias=rossie)
Date: 23.05.-20.06.2022
23.05. Monday (2:00-4:00 pm)
25.05. Wednesday (3:00-5:00 pm)
30.05. Monday (2:00-4:00 pm)
02.06. Thursday (2:00-4:00 pm)
07.06. Tuesday (2:00-4:00 pm)
09.06. Thursday (2:00-4:00 pm)
13.06. Monday (2:00-4:00 pm)
15.06. Wednesday (2:00-4:00 pm)
20.06. Monday (2:00-4:00 pm)
Location: Room 2298, AVZ (In special cases Zoom is possible as well)
Registration: Email to multiscale.clocks[at]uni-kassel[dot]de
Course objectives:
The goal of the course is to provide you a strategy for approaching a manuscript and also to provide insight into some technical details of both science and English writing construction that will help smooth your way. Therefore we expect everyone to work on a writing project, either a draft manuscript or a preliminary manuscript/outline for a research project in progress.
We anticipate there may be three types of writers in the course: those who have begun a manuscript already, those who are ready to begin writing a manuscript for research that is done or almost done, and those who know what their project is but have collected little or no data. All three types of writers are welcome. You should expect to devote 1-2 h of writing per day to make good progress on a new manuscript draft.
- If you begin with a manuscript draft, our hope is that you will be able to develop this draft significantly during this course.
- If you begin with all your data in hand and nothing written, developing a 1st draft for a manuscript is a reasonable project for this course. It will take work.
- If you are just beginning your project, you can develop an outline of a manuscript with figures and a summary of critical experiments, then work on this outline as you perform and review your research and talk to your advisor and colleagues.
This course meets for 2- 50 min sessions 2 days per week. In addition to course meetings, Mrs. Rossie offers to meet with each student seperately to individually provide detailed feedback on their writing project.
Lecturer: Dr. Natalia Filimonova
Date: 04.05.-25.05.2023
This seminar covered essential topics in statistical analysis and research methodology for biomedical journals. It explored the reliability and reproducibility of measurements, sources of variation, hypothesis testing, types of data, descriptive statistics, comparison of samples, correlation analysis, factor analysis, cluster analysis, and meta-analysis. The seminar aimed to provide participants with a comprehensive understanding of statistical concepts and techniques commonly used in biomedical research.
Lecture Contents:
1. Statistical modelling: Uniform requirements for manuscripts submitted to biomedical journals. Reliability and reproducibility of measurements. Variation and its sources. The relationship between validity and reliability. Shift (regression) to the mean. H0 and H1 hypothesis. Types of data. The normal distribution (Gaussian distribution). The central limit theorem. Tests of Normality (Kolmogorov-Smirnov test, Lilliefors test, Shapiro–Wilk test).
2. Descriptive Statistics: Mean, median, mode, standard deviation, variance, interquartile range. 95% Confidence interval of the mean. The frequency distribution. The visualization for quantitative and qualitative (ordinal and nominal) data.
3. Comparing two samples: Comparing two samples for independent and dependent quantitative normally, nonnormality distributed quantitative data and ordinal data: Student's t-test. Levene’s test. t-test with separate variance estimates. Mann-Whitney test, Wilcoxon test. The graphic representation of the results of the analysis.
4. A study of the factor's effect: Multiple comparisons. Bonferroni correction. Analysis of variance (ANOVA). Kruskal–Wallis H test or one-way ANOVA on ranks. Friedman's ANOVA test.
5. Analysis of the relationship for quantitative and qualitative (ordinal and nominal) data: Pearson correlation. Linear regression. Spearman's rank correlation. The graphic representation of the results of the correlation analysis. χ2 test. Fisher's exact test.
6. Factor and cluster analysis: Factor analysis as a method of reducing the number of variables and determining the structure of relationships between variables. Principal component analysis. Eigenvalues. Quality criteria for a factor model. Interpretation of the results of a factor model. The Anokhin theory of the functional systems. Cluster analysis as a method of Unsupervised learning in Machine learning.
7. Small sample: The power of the test. Sample size determination. Simpson's paradox. Stratification and standardization of data. Meta-analysis.
All lecture slides and recordings are available on moodle (https://moodle.uni-kassel.de/course/view.php?id=9713)
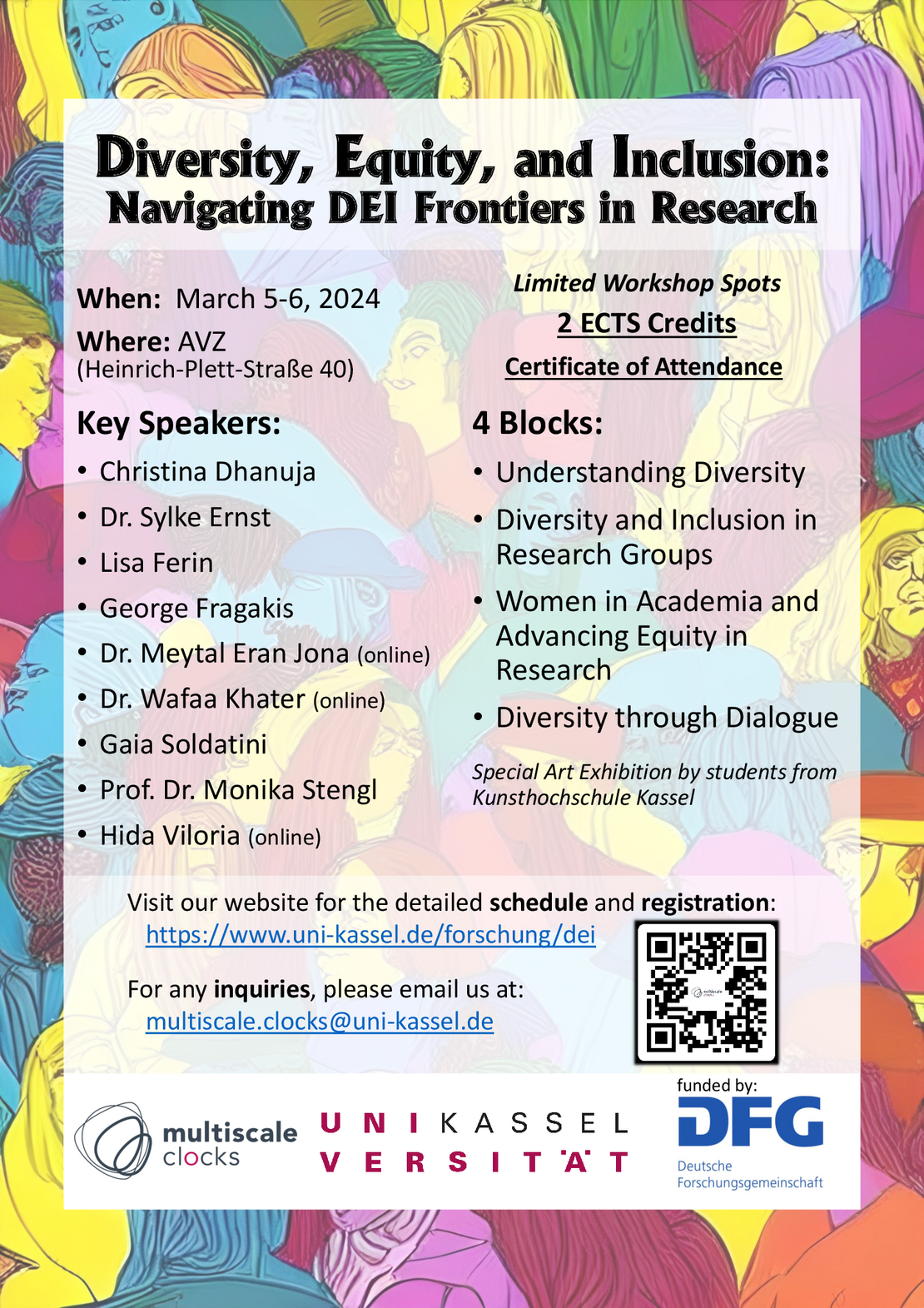
Navigating DEI Frontiers in Research
When/Where: March 5-6, 2024 / AVZ, Heinrich Plett Straße 40, 34132, Kassel
Event Highlights:
- Engaging discussions in four thematic blocks.
- Inspiring talks by renowned speakers.
- Interactive workshops.
- You can find a detailed program here.
Attendance is free of charge! Vegan food and coffee are included!
Language: English
Earn 2 ECTS Credits: Participants can earn 2 ECTS credits by attending the whole event in person, engaging actively, and submitting an 800-word essay afterward. Attendance is mandatory for ECTS credits.
Certificate of Attendance: For those who don't need ECTS credits, a Certificate of Attendance will be provided upon request, as long as at least 80% of the event is attended.
Questions or Inquiries: For any questions or inquiries, please reach out to us at multiscale.clocks[at]uni-kassel[dot]de.
List of artworks: diversity, equity, and inclusion
Chen Juejun: Mechanical Resonance, 2023 film, 04:14 min
This work emerges from gestures that appear in my body from time to time; they are foreign and familiar. When I trace these poses anchored in my bones, I see a particular form.
Anja Kellner: Revolution gegen Raster, 2024 animation, 00:10 min
Grid out, don't riot. The revolts also rasterize. Recursively grid societies in transition. Rastert.
Anna Metta, Robin Rösing: KOMMA kicken! 2023 animation, 04:30 min
KOMMA is a political education project with a focus on media education for girls and queer young people. The gender-sensitive and gender-homogeneous framework of the program offered the participants a safe space to try out a sport that is supposedly not "typically for girls" and enabled them to share an empowering experience. At the same time, the young people were able to reflect on gender stereotypes and their effects during the interviews shown in the film.
Marlene Rabe: custome-cake-swap, 2023 animation, 01:34 min
There are two wolves inside of you. One wants to expose the violence that lies in heteronormativity. The other one wants to destroy binary gender roles.
Seymoure Konnemann: Night Train, 2024 animation, 01:30 min
The animation "Night Train" is about the experience of waves of anxiety while being in public spaces, such as a train, as a gender-nonconforming person. I mostly mind my own business until some others start staring visibly longer than a normal glance, which causes fear in myself. In short, taking the train often causes me anxiety because I can't simply walk away if someone intends to cause harm.
Yu Xiaoxuan, Yang Tianshu: Starren, 2024 animation, 05:43 min
The "Starren" is a 5 minutes and 43 seconds 2D animation created by Tianshu Yang and Xiaoxuan Yu. It tells an everyday story about staring and being stared at. Being stared at by others in public can often be an uncomfortable experience. Even though we understand that not every gaze is meant negatively, we still feel discomfort or insecure. So what can we do about it?
Theo Lucas: astro plant boy 68, 2024 animation, 07:58 min
Pascalina Krummenauer: in vivo neona, 2023 animation, 11:14 min
With using multiple associative techniques on a mixed media base, the short film 'In Vivo Neona' tries to stay close and direct to the emotions felt in the process of it's creation.
Paula Berger: Gelbe Karten & Lila Latzhosen, die Filmkamera, Fundstücke der Borkener Frauenbewegung, 2023 film, 05:54 min
What do a doorknob, purple overalls and a movie projector have in common? They all tell stories from the Borken women's movement. Ten Borken women from two generations meet to talk about the history of these objects.
Anna Bellmann: Mother, 2023 performance (film documentation)
The work deals with the change, concealment and revelation of family history. The performance is dedicated to my great-great-grandmother, who, as a day laborer, raised an illegitimate child alone. As the father refused to recognize his son, she passed her own family name to her child - a huge scandal in her strictly catholic village. Although she is the founder of our family, her story was kept secret for generations and was only revealed 140 years later.
Herr v. Rehtanz: das Unbehagen – Vergegenwärtigte Fragmente, 2021 film, book
Under the title The Unease - Fragmentary Recollection, the artist Herr v. Rehtanz is showing an excerpt from their graduation project. They undertake an autobiographical retrospective in which they reflect on their experience as a queer person with a history of migration in Germany.